This baby with a head camera helped teach an AI how kids learn language
A neural network trained on the experiences of a single young child managed to learn one of the core components of language: how to match words to the objects they represent.
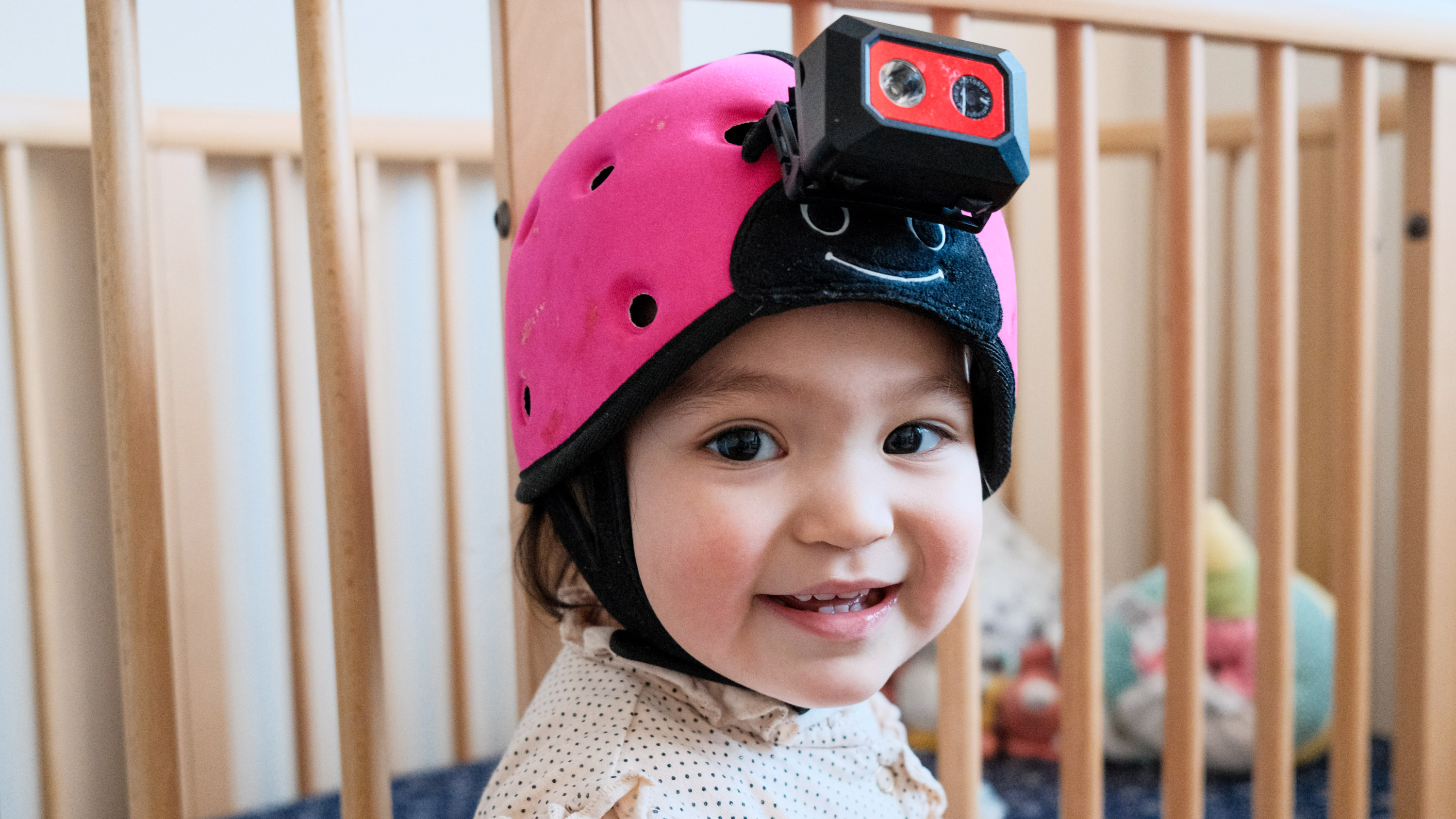
Human babies are far better at learning than even the very best large language models. To be able to write in passable English, ChatGPT had to be trained on massive data sets that contain millions or even a trillion words. Children, on the other hand, have access to only a tiny fraction of that data, yet by age three they’re communicating in quite sophisticated ways.
A team of researchers at New York University wondered if AI could learn like a baby. What could an AI model do when given a far smaller data set—the sights and sounds experienced by a single child learning to talk?
A lot, it turns out. The AI model managed to match words to the objects they represent. “There’s enough data even in this blip of the child’s experience that it can do genuine word learning,” says Brenden Lake, a computational cognitive scientist at New York University and an author of the study. This work, published in Science today, not only provides insights into how babies learn but could also lead to better AI models.
For this experiment, the researchers relied on 61 hours of video from a helmet camera worn by a child in Australia. That child, Sam, wore the camera off and on for one and a half years, from the time he was six months old until a little after his second birthday. The camera captured the things Sam looked at and paid attention to during about 1% of his waking hours. It recorded Sam’s two cats, his parents, his crib and toys, his house, his meals, and much more. “This data set was totally unique,” Lake says. “It’s the best window we’ve ever had into what a single child has access to.”
To train the model, Lake and his colleagues used 600,000 video frames paired with the phrases that were spoken by Sam’s parents or other people in the room when the image was captured—37,500 “utterances” in all. Sometimes the words and objects matched. Sometimes they didn’t. For example, in one still, Sam looks at a shape sorter and a parent says, “You like the string.” In another, an adult hand covers some blocks and a parent says, “You want the blocks too.”
The team gave the model two cues. When objects and words occur together, that’s a sign that they might be linked. But when an object and a word don’t occur together, that’s a sign they likely aren’t a match. “So we have this sort of pulling together and pushing apart that occurs within the model,” says Wai Keen Vong, a computational cognitive scientist at New York University and an author of the study. “Then the hope is that there are enough instances in the data where when the parent is saying the word ‘ball,’ the kid is seeing a ball,” he says.
Matching words to the objects they represent may seem like a simple task, but it’s not. To give you a sense of the scope of the problem, imagine the living room of a family with young children. It has all the normal living room furniture, but also kid clutter. The floor is littered with toys. Crayons are scattered across the coffee table. There’s a snack cup on the windowsill and laundry on a chair. If a toddler hears the word “ball,” it could refer to a ball. But it could also refer to any other toy, or the couch, or a pair of pants, or the shape of an object, or its color, or the time of day. “There’s an infinite number of possible meanings for any word,” Lake says.
The problem is so intractable that some developmental psychologists have argued that children must be born with an innate understanding of how language works to be able to learn it so quickly. But the study suggests that some parts of language are learnable from a really small set of experiences even without that innate ability, says Jess Sullivan, a developmental psychologist at Skidmore University, who was part of the team that collected Sam’s helmet camera data but was not involved in the new study. “That, for me, really does shake up my worldview.”
But Sullivan points out that being able to match words to the objects they represent, though a hard learning problem, is just part of what makes up language. There are also rules that govern how words get strung together. Your dog might know the words “ball” or “walk,” but that doesn’t mean he can understand English. And it could be that whatever innate capacity for language babies possess goes beyond vocabulary. It might influence how they move through the world, or what they pay attention to, or how they respond to language. “I don’t think the study would have worked if babies hadn’t created the data set that the neural net was learning from,” she says.
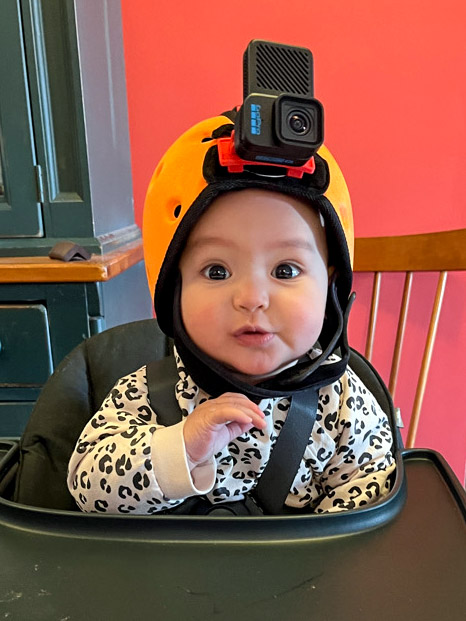
The next step for Lake and his colleagues is to try to figure out what they need to make the model’s learning more closely replicate early language learning in children. “There’s more work to be done to try to get a model with fully two-year-old-like abilities,” he says. That might mean providing more data. Lake’s child, who is now 18 months old, is part of the next cohort of kids who are providing that data. She wears a helmet camera for a few hours a week. Or perhaps the model needs to pay attention to the parents’ gaze, or to have some sense of the solidity of objects—something children intuitively grasp. Creating models that can learn more like children will help the researchers better understand human learning and development.
AI models that can pick up some of the ways in which humans learn language might be far more efficient at learning; they might act more like humans and less like “a lumbering statistical engine for pattern matching,” as the linguist Noam Chomsky and his colleagues once described large language models like ChatGPT. “AI systems are still brittle and lack common sense,” says Howard Shrobe, who manages the program at the US government’s Defense Advanced Research Projects Agency that helped fund Lake’s team. But AI that could learn like a child might be capable of understanding meaning, responding to new situations, and learning from new experiences. The goal is to bring AI one step closer to human intelligence.
Update: We removed a reference to the city that Sam lives in at his parents' request.
Deep Dive
Biotechnology and health
The next generation of mRNA vaccines is on its way
Adding a photocopier gene to mRNA vaccines could make them last longer and curb side effects.
An AI-driven “factory of drugs” claims to have hit a big milestone
Insilico is part of a wave of companies betting on AI as the "next amazing revolution" in biology
Ready, set, grow: These are the biotech plants you can buy now
For $73, I bought genetically modified tomato seeds and a glowing petunia.
The quest to legitimize longevity medicine
Longevity clinics offer a mix of services that largely cater to the wealthy. Now there’s a push to establish their work as a credible medical field.
Stay connected
Get the latest updates from
MIT Technology Review
Discover special offers, top stories, upcoming events, and more.